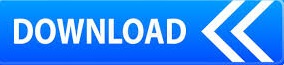



, 2005 Schölkopf and Smola, 2002 ) however, in practice many researchers prefer simpler algorithms ( Hand, 2006 ). Relatively, sophisticated methods such as penalized regression, support vector machines, random forests, bagging and boosting have seen detailed treatments in the statistics and machine learning literature ( Bühlmann and Van De Geer, 2011 Hastie et al. The successful translation of genomic signatures into clinical settings relies on good discrimination between patient subgroups that should receive different clinical management. It performs no worse, and sometimes better, than popular and much more CPU-intensive methods for discrimination, including lasso and ridge regression.Īvailability and Implementation : Más-o-menos is implemented for survival analysis as an option in the survHD package, available from and submitted to Bioconductor. We find that despite its simplicity, más-o-menos can achieve good discrimination performance. We study its behavior theoretically, in simulations and in an extensive analysis of 27 independent gene expression studies of bladder, breast and ovarian cancer, altogether totaling 3833 patients with survival outcomes. Results : We give a precise definition of a popular simple method we refer to as más-o-menos, which calculates prognostic scores for discrimination by summing standardized predictors, weighted by the signs of their marginal associations with the outcome. However, few simple algorithms have been formally described or systematically investigated. Many sophisticated algorithms have been proposed in the statistics and machine learning literature, but in practice simpler algorithms are often used. Motivation : The successful translation of genomic signatures into clinical settings relies on good discrimination between patient subgroups.
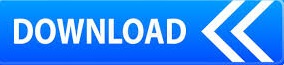